For a retail store, Black Friday is unlike any other day of shopping all year. In-store, online, small boutiques, large chains, Black Friday sales are every retailer’s harbinger for the holiday buying season and annual earnings and whether sales numbers will hit their mark.
Call it a day of reckoning to gauge a retailer’s success. Or even their survival. And to make decisions that spur customer spending, understanding customer behaviors and buying patterns is never more critical for retail management than on Black Friday.

“A machine learning problem”
“Trying to figure out how to drive and manage and create more specialized and unique customer experiences is absolutely a machine learning problem,” according to Bob Hetu of Gartner. Hetu is Gartner’s retail research director, and while he doesn’t tie customer behaviors and buying habits entirely to Black Friday, the day after Thanksgiving certainly intensifies his logic:
If leaders in retail management understand why customers behave the way they do, Hetu says, machine learning can help retailers influence that behavior more impactfully.
For instance, retailers can use a machine learning model to cross reference a customer’s past purchases and the price of those products with new product information. Data for the customer’s behavioral drivers help retailers define the person’s buying persona and identify trends in their shopping behavior for purchases in the future.
Larger scale, a machine learning model can detect trends for a product favored by entire consumer communities, say a certain make and version of a smartphone or other mobile device. Think of all the electronics that a retail store trots out for Black Friday, and machine learning can more accurately determine which products would be best-sellers.
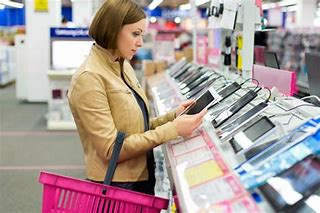
Before machine learning
Prior to using machine learning and AI at the levels they do today, retailers generally tracked consumer shopping habits and market trends using tools like CRM (for customer purchase histories), loyalty programs, social media posts, and industry reports (consumer demand). Enter the use of data analytics and machine learning in a more sophisticated manner, a move that paid off early on.
In 2016, for instance, research from McKinsey & Company showed that U.S. retail supply chain operations had increased their operating margin by nearly one-fifth over a 5-year period after putting a greater emphasis on data analytics. Ever since, the key for retailers has been to use data to even greater effect, and Black Friday is the ultimate proving ground.
At this point, machine learning and larger volumes of data let retailers predict buying behavior with a much higher degree of accuracy. Retail management decision-makers are better equipped to understand what products consumers want, how much they’re willing to pay for them, and whether a shopper is “just looking” or ready to make a purchase. Data analytics further enable retailers to ensure product supply and availability.
So rather than estimated guesses based on social media threads or CRM records that might or might not be up to date, machine learning enables retailers to understand customer behavior based on intelligent data analytics.
To help make the customer’s experience more enjoyable, machine learning principles can identify and assess the actions of shoppers and associates throughout a retail store. Does a customer have to crouch down or reach overhead to get a product off the shelf? Are display racks placed where products are readily visible?
As Gartner’s Hetu puts it, the uppermost advantage of machine learning is its ability to easily analyze and create prediction models based on a significant amount of data. “This could include data about sales, customers, products, employees, vendors and more.”
Predictive and prescriptive analytics
Authors at Oliver Wyman note that retailers have historically tried to predict sales by applying business logic based on experience — “the quality of a brand, the shelf placement, the promotion, and so on.” To get forecasts for a shopping event such as Black Friday, decision makers in retail management have routinely used a series of linear regressions to plot known sales volume against the variables. The downside of this approach is that it relies on humans to select and analyze data, which can jeopardize forecasting accuracy.
With machine learning and data models for predictive and prescriptive analytics, however, retailers can look at history to determine critical data factors and safely predict what will occur based on a much larger set of variables. Here’s the gist in a retail framework:
For insight, predictive analytics can help a retail chain predict what will happen based on a its current circumstances; that is, what will happen if existing organizational practices stay as they are. A predictive model uses the same type of data as descriptive analytics — customer comments, product and services reviews, website traffic and so on — which enable a retail organization to assess its current circumstances by analyzing records of past events. Predictive models can include descriptive outcomes themselves.
For foresight, instead of predicting an outcome, prescriptive analytics let retailers tweak specific variables to achieve the best possible result and then prescribe the course of action accordingly. IBM further defines prescriptive analytics as consisting of optimization, or how to achieve the best outcome, and stochastic optimization, or how to achieve the best outcome by accounting for uncertainty in existing data in order to make better decisions.
Therefore in the ML forecasting world, retail management leaders can build predictive data analytics models in which machine learning algorithms randomly create thousands of decision trees based on sub-groups of explanatory variables. The algorithms then combine these thousands of trees to create a single predictive model incorporating all the variables.
Especially for sales promotions like those for Black Friday, once a predictive algorithm is “trained,” it can automatically, and more accurately, predict sales at the product level. The model continues to learn as it ingests larger amounts of data and processes results. Or for a more futuristic view of the retail landscapes, prescriptive analytics can help retailer achieve a best-case result and determine the best course of action.
Machine learning, AI and retail in the near future
Here are some things on the machine learning and AI horizon for retail, and some that are already available — just in time for Black Friday.
- AI and machine learning will soon reach the point of being able to suggest marketing actions and promotions in a retail store. For example, placing a best-selling product more prominently than lesser-selling competitive brands, suggesting discounts for the brands that aren’t selling, and clearing inventory and shelf space for better selling products.
- Instead of using cameras only for security, a retail store will use them for computer vision that requires little or no human intervention to monitor video footage and gather correlating data. Solutions such as Aurora from RetailNext are already available to process marketing analytics from video data. The Aurora device enhances data for customer traffic using machine vision to gather data for demographics, customer engagement, and other retail store metrics in real time.
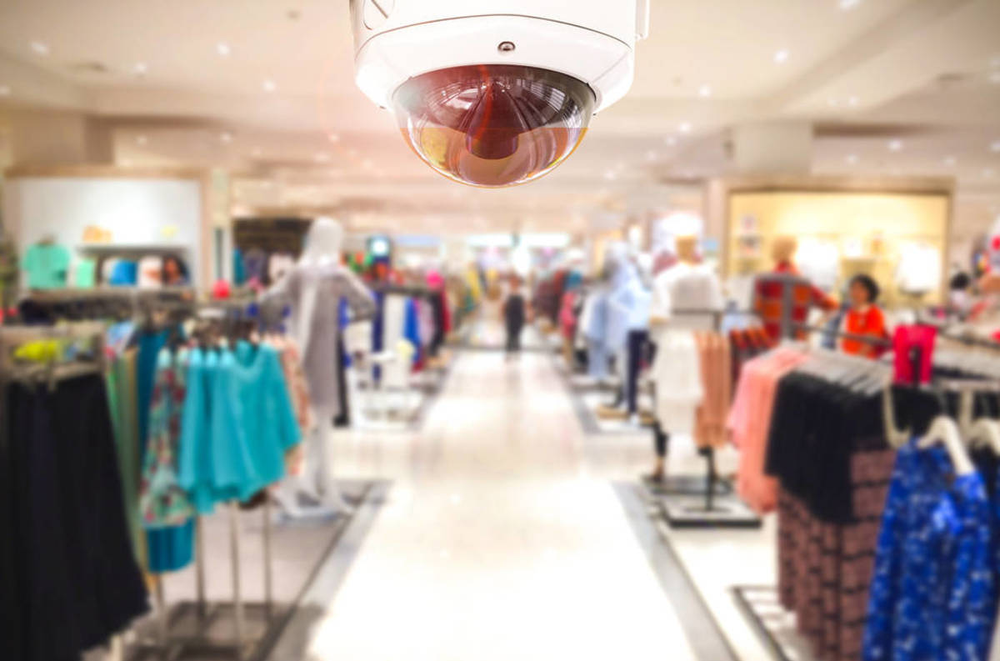
- In-store retail environments will incorporate more sensors to track customer behaviors and associated information, allowing retailers to encourage sales and cart value in new ways. For instance, cameras could detect the walking patterns of customers and the products they stop to focus on. Retail management could then analyze overall customer interest in various products and restructure their retail store layouts accordingly. Retail chains could additionally test new products in locations that get maximum foot traffic and visual attention from shoppers.
- In-store digital shopping assistants will continue to become more commonplace, and machine learning will help devices gradually become more intelligent with cognitive tools to receive input (customers’ questions and inquiries) and issue answers in natural language. Beyond current chatbot technologies, smartphones employing this capability could well become the chosen “sales assistant” for customers in a physical retail store environment. Macy’s has already taken this step for its customers, having partnered with IBM’s Watson more than a year ago to launch the mobile-based Macy’s On Call shopping assistant platform. The AI-powered platform uses Watson’s Natural Language Classifier API, and even provides bilingual support for Spanish-speaking customers.
- To predict inventory needs in real time, machine learning will enable retail management to do so using a combination of real-time, historical, online and offline customer purchase data. This will improve inventory control based on various factors like the shopping season, the day of the week, activity at nearby stores, online shopping activity from customers in the same geographic area, and so on. This has Black Friday written all over it.
- Relatedly for inventory, machine vision technology — for cameras installed throughout a retail store as well as hand-held cameras for employees — will be able to generate accurate, real-time data for entire product inventories in a specific store. Such an approach could detect unusual patterns in inventory data, particularly an uptick in sales of a certain product that would warrant a faster re-order than perhaps planned. AI systems could then be relied on to make product orders automatically, without human approval. Again, a real benefit for shopping extravaganzas such as Black Friday.
- For online retail businesses, beyond detecting clicks, scrolls and user behavior, machine learning will enable them to feed this instrumented information into data processes that can more accurately predict the success (or failure) of online advertising efforts in real time.
Final thoughts
For retailers of every shape and size, technologies like AI, machine learning and machine vision are shifting retail stores and operations towards an instrumented state. And in moving away from how things “have always been done,” retail management leaders are moving toward using data to make decisions in real-time that will help them adjust to customer behaviors, make far more accurate sales forecasts, control inventories more effectively, and even alter product displays to cut losses and produce sales gains.
Especially with a retail store becoming more like a website for shoppers, retailers that don’t implement technologies to track, collect, analyze and use data to improve operations and the customer experience will need to rethink their approach — and soon.
Black Friday is just around the corner.
Contact us
ClearObject knows all about machine learning solutions. In addition to IoT Engineering, Analytics and Connected Product Development, we’re experts at developing and implementing targeted data analytics strategies to get the most value from your data, including deploying and managing serverless cloud solutions for AI tools and end-to-end machine learning models.
#blackfriday #blackfridaysales #retailstore #retailmanagement #machinelearning #machinelearningalgorithms #dataanalytics